Introduction to Data Query Engines
- Asher T
- Apr 18, 2022
- 3 min read
Updated: May 1, 2022
Big Data is one of the most valuable commodities in business today, but only if organizations have the power to analyze it and make it work for them.
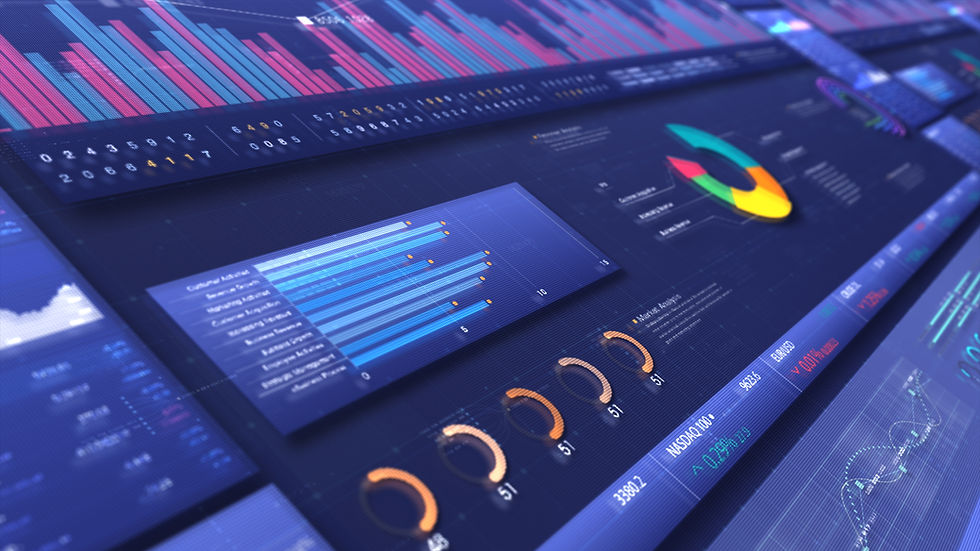
The term “Big Data” represents a massive amount of structured and unstructured data from several different sources. As more and more companies find themselves in possession of Big Data, there’s a greater need for tools that can extract useful insights from their monstrous reservoirs of information.
Data query engines are one of the most valuable tools in this category. In a nutshell, query engines allow companies to connect data from any source, any technology, or in any format and then query it with simple SQL commands.
In this high-level overview, we’ll take a look at the power of data query engines, as well as provide a few tips for implementing them.
Why Use Query Engines?
To make use of their Big Data, organizations need a way to query, merge, and join data seamlessly, but the challenge is the sheer amount of different data sources and formats.
Data is found in relational databases, CSV files, XML spreadsheets, text files, non-SQL databases, and several other sources, each of which has a completely different format and structures, making it extremely difficult to analyze.
The old classic solution is to upload all of this unstructured data to a single relational database, but this requires a lot of scripts and ETL (extract, transform, and load) programs to deal with the many different formats. Relational databases are also quite slow when it comes to processing data as they don’t usually have the computing power to deal with many sources.
In order to extract any meaningful information from these data sources, companies need them to fall under a single common format, which is where data query engines come in. Query engines allow companies to connect data from different sources in different formats and different technologies and then query that data in the same way.
All query engines work with SQL, a data query language that is well-known and easy to learn. As a widely used and accessible query language, SQL is the defacto standard for commanding a system on how to display data. Query engines offer the standard SQL interface while hiding the complexity of the data storage configuration, making them extremely valuable and easy to use.
Distributed Power
Data query engines are distributed in a way that allows organizations to process Big Data extremely quickly.
Relational databases are usually configured to one node, host, or server. Their performance is determined by how much memory or processing power they have access to. Increasing computational power to improve the performance of a relational database is known as vertical scalability, which is an expensive process.
In Big Data, there is a more powerful approach known as distributed computing, which involves implementing a cluster of computers or servers that work together to solve a problem. All data query engines are distributed based on this approach, mostly with a driver node in command of the computing power, a resource manager for administering work between nodes, and a group of worker nodes that perform the computations.
With this architecture, companies can get much better response times for queries than are possible with a simple relational database.
Tips and Challenges
As we’ve seen from the architecture examples above, installing a query engine can be challenging for some companies and the learning curve is slightly steeper than with relational query engines.
Comments